Last year TfL trialled real-time AI analysis of CCTV footage at Willesden Green station. In the past few days the results of FOI requests by journalists have come through and the details of the scheme can be revealed:
takes.jamesomalley.co.uk
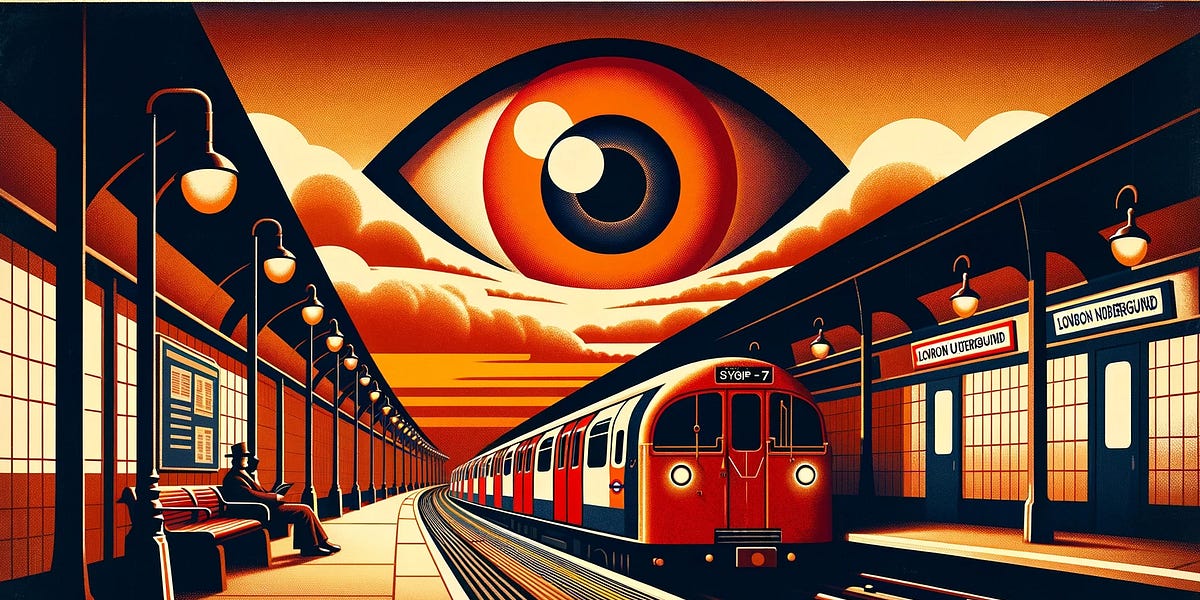
TfL's AI Tube Station experiment is amazing and slightly terrifying
Mind the Orwellian Surveillance Apparatus

The experiment began in October 2022 and ran until the end of September last year. Willesden Green was selected specifically because it was the sort of station that could benefit from an extra pair of eyes: It’s classed as a small “local” station, and it does not have step-free access. The reason why this matters will soon become clear.
To make the station “smart”, what TfL did was essentially install some extra hardware and software in the control room to monitor the existing analogue CCTV cameras.
This is where things start to get mind-blowing. Because this was not just about spotting fare evaders. The trial wasn’t a couple of special cameras monitoring the ticket gate-line in the station. It was AI being applied to every camera in the building. And it was about using the cameras to spot dozens of different things that might happen inside the station.
For example, if a passenger falls over on the platform, the AI will spot them on the ground. This will then trigger a notification on the iPads used by station staff, so that they can then run over and help them back up. Or if the AI spots someone standing close to the platform edge, looking like they are planning to jump, it will alert staff to intervene before it is too late.
In total, the system could apparently identify up to 77 different ‘use cases’ – though only eleven were used during trial. This ranges from significant incidents, like fare evasion, crime and anti-social behaviour, all the way down to more trivial matters, like spilled drinks or even discarded newspapers.
[...]
What’s amazing about this is that it provides an amazingly granular level of detail over what is happening at the station – and that it’s only possible because AI computer vision essentially changes what used to be static images into a series of legible digital building blocks that logic can be applied to.
For example, in the “safeguarding” bucket of use-cases, the AI was programmed to alert staff if a person was sat on a bench for longer than ten minutes or if they were in the ticket hall for longer than 15 minutes, as it implies they may be lost or require help.
And if someone is stood over the yellow line on the platform edge for more than 30 seconds, it similarly sends an alert, which prompts the staff to make a tannoy announcement warning passengers to stand back. (There were apparently 2194 alerts like this sent during the trial period – that’s a lot of little incremental safety nudges.)
Helping people is one thing, but the system is also capable of spotting different sorts of bad behaviour too.
For example, if someone passes through the station with a weapon, the AI is apparently capable of spotting it. In fact, to train the system, TfL says it worked with a British Transport Police firearms officer, who moved around the station while wielding a machete and a handgun, to teach the cameras what they look like.
During the trial period there were apparently six ‘real’ weapons alerts. Though it’s not clear whether or not they were false positives, amusingly that’s more than the 4 alerts for smoking or vaping in the station.
My favourite thing from the TfL docs though is on the attempts by the AI to spot “aggressive behaviour”. Unfortunately for reasons TfL redacted from the documents, there was insufficient training data to make this reliable (what would this even look like?).
So TfL hit upon a novel solution: They instead trained the system to spot people with both arms raised in the air – because this is thought to be a “common behaviour” linked to acts of aggression (presumably raised fists or ‘hands up’ as a surrender-like gesture).
[...]
To make it possible for the cameras to spot people jumping the barriers, crawling under the barriers (!), or ‘tailgating’ – when multiple people pass through at the same time – TfL manually scrubbed through and tagged “several hours” of CCTV footage to train the system to teach it what fare evasion looks like.
And it appears the system got pretty good at spotting it.
Here’s one chart showing 3802 fare evasion alerts over the course of June 2023 – there were over 26,000 during eleven months of the trial in total.
Amusingly, to make detections more accurate, TfL had to go back and reconfigure it to avoid counting kids as fare evaders – and they did it by automatically disregarding anyone shorter than the ticket gates.
So TfL can seemingly identify fare evaders, but the agency also learned that stopping them may be harder. For a start, during the trial staff were not sent fare evasion alerts on their iPads deliberately. This was because (after discussions with the trade union) it was decided that it could put staff in a dangerous position if they’re supposed to confront, say, someone who just jumped a barrier.
But the bigger problem was, well, identifying suspected evaders. When the trial first started, all faces were automatically blurred to maintain passenger privacy – but when it moved on to phase 2, an exception was made for fare evasion.
This meant that the AI could feed into what was previously a manual process which would rely on station staff recognising repeat offenders, and then filing a manual report to TfL’s revenue enforcement team.
With AI however, this process can be automated, but only to an extent. The documents explain that recognised faces are manually double-checked for matches. Once they have been identified, TfL’s Revenue Control team then need to manually put together a case against the evader.
According to the above diagram, Revenue Control will try to determine patterns of behaviour when there is a persistent evader – and if they’re turning up to the station and skipping through in a reliable pattern, the inspectors will turn up at the station to ambush them – and then present them with all of the evidence against them.
However, exactly how helpful AI is here remains to be seen, as the report says that “The process was successfully tested and although we did not see the results we had hoped, we also ran out of time.”